Not another chatbot: Notes from a week with some of the top AI builders, platforms and innovators.
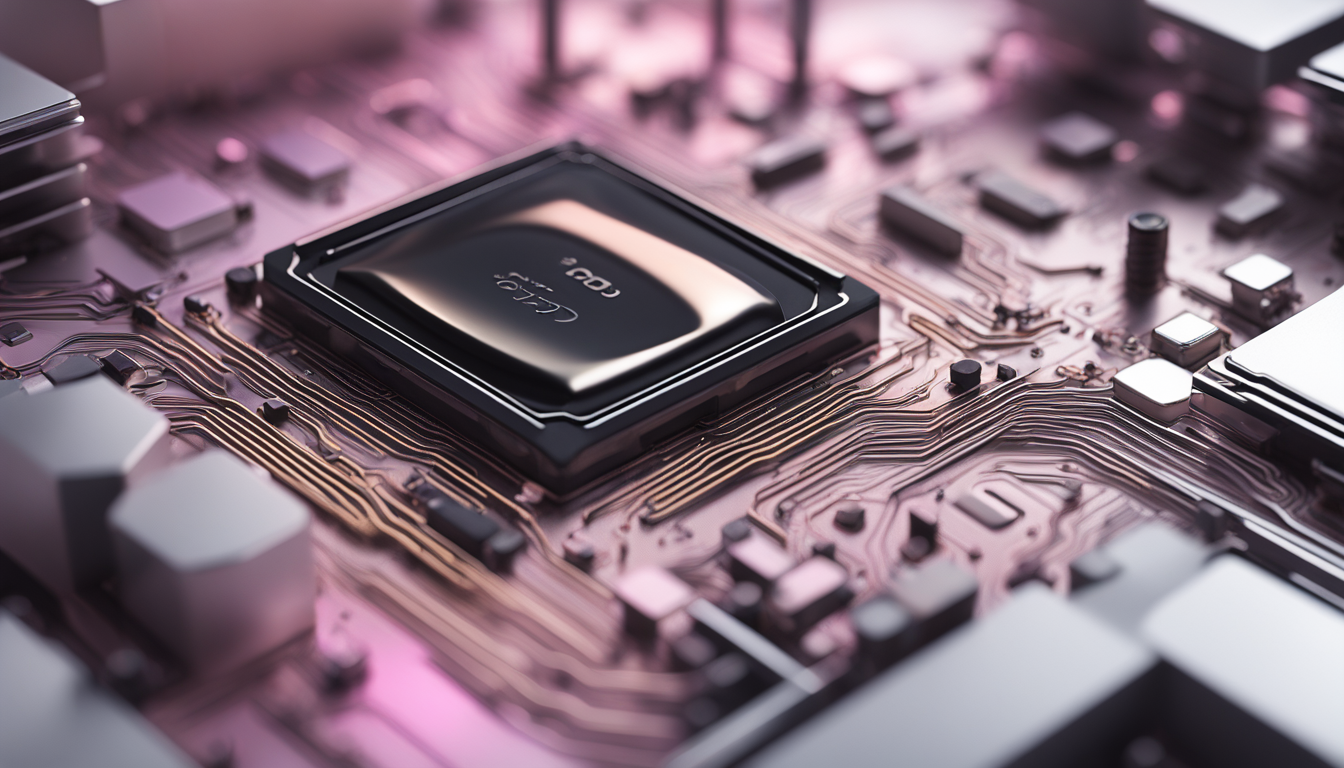
After a thought-provoking week in Silicon Valley connecting with pioneers in artificial intelligence, a few key themes emerged that will shape the future of AI. Trust, task focus, and workforce augmentation rose to the top as crucial considerations.
The Theory of Mind Debate
The week started off with a fascinating presentation by Dr. Kira Radinsky on the emergence of theory of mind in large language models. She overviewed research by Kosinski and others showing LLMs spontaneously developing abilities to infer beliefs, desires, and perspectives. This hints at human-level intelligence.
However, as noted in discussions led by Dr. Radinsky, researchers like Sap and Ullman argue LLMs still lack robust social intelligence. The rapid pace of advances makes assessing capabilities difficult. What’s clear is that LLMs display an increasing awareness of mental states, though debate continues over the extent of this emergent theory of mind.
The socio-technical implications of AI attaining theory of mind are profound. On one hand, it can enable empathetic interfaces and assistants. But it also further blurs the line between human and machine cognition. Responsibly shaping this technology is an urgent priority.
Trust and Explainability
With AI permeating high-stakes domains like finance, healthcare, industrial, energy, legal, education, establishing trust is paramount. The leading AI teams emphasized comprehensive guardrails to ensure reliable, ethical outputs. Extensive testing, safety procedures, and human oversight enable the safe deployment of powerful models.
Explainability also fosters trust. While large language models can generate remarkable text and insights, being able to understand the rationale behind the output is critical. AI developers are focused on providing transparency into model behavior through various methods of explanation.
On the trust front, a focus on quality also ensures trust. Builders in Generative AI need to focus on quality before price and speed. Having the fastest on-prem LLM is not what we should be shooting for this early into the process.
The Deeper the Task, The Higher the Value
Rather than building broad horizontal AI platforms, many innovators are diving deep into distinct valuable tasks. “Not another chatbot” read the t-shirt of a Japanese founder, reflecting the narrative of the week.
By specializing, startups can achieve greater accuracy and usefulness but also place an emphasis on the customer’s experience. Gathering insights from LLM interactions with your customer is a very new analytics collective we’ve never seen before.
As AI becomes more capable, a proliferation of deep vertical AI solutions tailored to specific tasks will emerge.
The Dawn of the AI Workforce
Automating repetitive workflows through AI agents will transform businesses. Relevance AI, an Australian startup enables anyone to build an AI workforce by combining modular building blocks. Agents equipped with the right skills, tools, and knowledge can take over manual processes across departments. While AI won’t replace human jobs entirely, it will drastically augment productivity. Gartner predicts that by 2030, 45% of labor hours will be automated. The future of work is already here.
Domain Specific Language for Planning Tasks
One limitation noted of current LLMs is poor performance on planning and reasoning tasks.
This is an area where domain-specific language (DSL) shows promise. Rather than using broad natural language training, DSLs provide a constrained vocabulary tailored to a specific task. Early research indicates DSLs can enable LLMs to successfully tackle planning challenges like scheduling, forecasting, travel booking, process optimization, and even building training data on past events. Though narrow in scope, DSLs unlock capabilities not readily achieved through general language models alone.
Taking on Nvidia
With the surge in generative AI adoption, demand for specialized AI hardware like GPUs is reaching all-time highs. Silicon Valley companies are exploring alternatives like Groq’s LPU chips which promise faster throughput for running large language models. Though still early, custom AI chipsets may eventually provide the infrastructure needed to scale deployment of LLMs across industries.
AI Infrastructure and Frameworks
The rapid advancement of generative AI is enabled by specialized infrastructure tailor-made for large language models. Companies like Groq offer dedicated AI chips while Anyscale provides a scalable serverless compute platform. Frameworks like Ray, created by Anyscale, simplify distributed training and deployment of GenAI models. These backend solutions overcome hardware constraints and complexity barriers that previously bottlenecked model development. With ready-made tools for training, optimizing, and running massive models, engineers can focus more on novel applications rather than infrastructure. The right platforms, frameworks, and chips provide the foundation for organizations to build impactful GenAI responsibly and efficiently.
In addition to these key themes, several other AI trends came up repeatedly in our discussions:
The Smaller the Better…
There is growing emphasis on smaller, specialized models rather than massive general models trained on all data. More focused architectures improve relevance and reduce computing costs.
AI Observability
A key enabler of trust is observability of model performance and behavior after deployment. Companies like Maxim and Fiddler provide ML testing and monitoring solutions tailored to generative AI that enable continuous tracking of quality metrics and model drift. This empowers organizations to identify reliability issues and make informed optimizations to improve robustness. Comprehensive observability frameworks are critical infrastructure for responsible scaling of LLMs.
Multimodal’s Moment
Combining multiple data types like text, images, and video in unified models unlocks new valuable applications across industries.
Opensource LLMs
While large proprietary models receive most attention, open source LLMs are rapidly advancing as well. These publicly available models like Meta’s LLaMA, Mistral, and Hugging Face’s Bloom enable wider access and community-driven innovation. As capabilities improve, interest and adoption of open source LLMs will likely grow, especially among startups and academia. Though still lagging commercial offerings, they provide low-cost options and customization potential. The open ecosystem facilitates model reproducibility and transparency too. In the long run, open source LLMs may challenge the dominance of restricted access models. Community development and evaluation could become vital to responsible progress in AI.
Surging Compute Costs
As demand for complex AI systems grows, compute remains a major cost. Cloud platforms like AWS facilitate optimization, allowing easy switching between models based on speed, accuracy, and price. But even more impactful are software efficiencies - sharing compute across applications, multiplexing queries, and rightsizing inference models. With careful architecture, the exponential power of LLMs can scale affordably. Cost-conscious engineering is key; the fastest or largest model alone does not guarantee utility. Keeping compute flexible, not overprovisioned, saves resources for higher-value efforts.
Ideological Implications
As AGI appears more plausible, humanity will reconsider core economic and political structures incompatible with machine intelligence.
Silicon Valley continues to push the boundaries of artificial intelligence. It was thought-provoking to connect with the minds shaping the next paradigm of AI productivity, while directly confronting the hard questions around trust and ethics. The overarching goal is leveraging AI to expand human potential. With responsible leadership from pioneering companies, the opportunities ahead are boundless.