Game Theory and AI: Leveling the Investing Playing Field
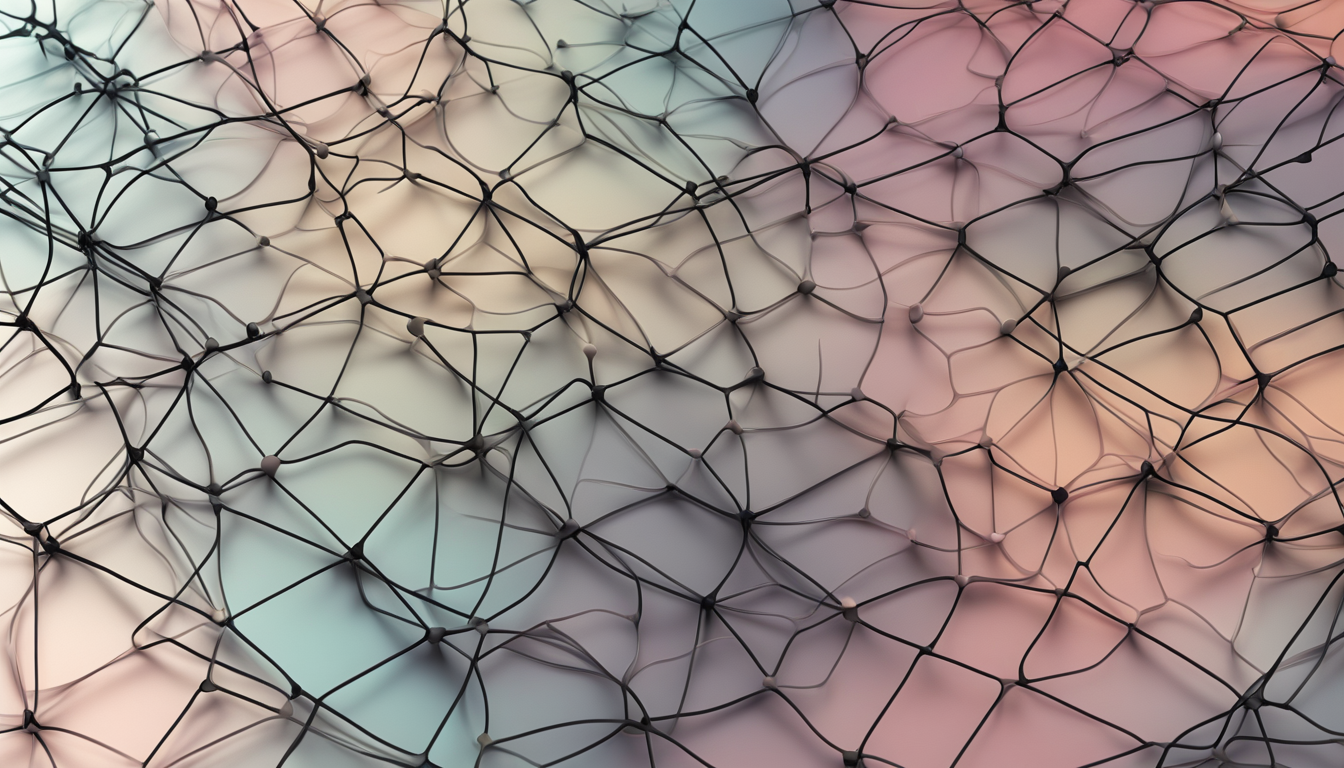
Game theory has provided a useful framework for understanding strategic decision-making for over 70 years. As AI becomes increasingly integrated into finance, game theory principles can help ensure these systems make fair and balanced recommendations. In this post, we’ll explore how AI and game theory intersect for investing.
The Basics of Game Theory
Developed in the 1940s by mathematician John von Neumann, game theory examines how rational decision-makers interact in strategic situations. It has been applied to fields like economics, political science, and computer science.
As outlined in the book “Game Theory and Macro Investing Playbook,” game theory involves analyzing:
- The objectives of each player, shaped by their culture and incentives
- The bargaining power of each player based on resources they control
- The possible modes of action and credibility of threats
By considering these factors, game theory provides a framework for anticipating potential actions and reactions. A classic example is the prisoner’s dilemma where two suspects must choose whether to confess or stay silent.
Applications in Investing
Game theory is well-suited for analyzing complex geo-political scenarios that impact markets. As head of William Blair’s Dynamic Allocation Strategies Team Brian Singer explains:
“Game theory provides boundaries for the complexity of the political and geopolitical macro developments to be analyzed…The focused, even confining, discipline of game theoretical models afford context within which we can fill knowledge gaps with independent, seasoned judgment in order to comprehend, embrace, and exploit inevitable uncertainty.”
Rather than assuming efficient markets, game theory makes it possible to model complex negotiations between countries that influence asset prices and portfolio allocations.
The Rise of LLMs
Recent breakthroughs in large language models (LLMs) like GPT-4 have opened up new possibilities for applying game theory with AI. LLMs can understand and generate nuanced natural language. This makes them uniquely suited for modeling complex strategic scenarios.
LLMs can ingest research papers, news articles, speeches, and other textual data to identify relevant players, objectives, and actions. For example, an LLM could analyze a transcript of an earnings call to determine a company’s priorities and potential moves.
LLMs can also simulate negotiations by taking on the persona of different players and predicting responses. This type of role playing allows an AI system to model hypothetical scenarios and gain insights into how situations may unfold.
By leveraging the knowledge and reasoning capabilities of LLMs, game theory AI can better comprehend the incentives and relationships driving strategic decision making. LLMs enable more nuanced modeling that accounts for subtleties in language and human psychology.
While LLMs have shortcomings like potential bias, their flexible linguistic skills make them a promising tool for applying game theory. LLMs can parse complex dynamics from text and generate persuasive narratives to reason about strategic scenarios. With oversight and principle-based design, LLMs have the potential to model game theory situations with greater sophistication.
Multi-Agent Reinforcement Learning
An emerging AI technique called multi-agent reinforcement learning (MARL) combines game theory with reinforcement learning’s trial-and-error approach.
With MARL, AI agents can gain experience through repeatedly simulated scenarios. The agents iteratively improve their strategies over many simulations in response to the evolving strategies of other players.
Running trained simulations provides several key benefits:
- Agents can explore a wider range of scenarios than real-world data permits. For example, an agent could simulate a novel virus outbreak that rapidly spreads globally, disrupting supply chains and labor markets. This allows preparation for potential future pandemics beyond historical precedents.
- Repeated simulations compound knowledge as agents encounter rare edge cases. One simulation may involve a major cyberattack shutting down a nation’s power grid, while another introduces a massive earthquake in a key manufacturing hub. Exposure to myriad edge cases makes agents more robust.
- Agents can uncover “black swan” events through extensive permutations. By combining different extreme weather events, geopolitical crises, and economic variables in novel ways, agents may encounter unlikely but high-impact scenarios. This allows identification of invisible tail risks.
- Strategies are polished through low-risk practice. Before being deployed in real-world situations, agents refine techniques through thousands of simulations. For example, reacting to the sudden death of a key political figure or responding to a surprise restriction of a critical export. This builds strategic experience before consequences matter.
Large language models can enhance these simulations by dynamically generating new scenarios with reasonable yet novel parameters. The permutations are endless.
Over many iterative simulations, the MARL agents grow smarter and more strategic in their reactions and negotiations. The combination of reinforcement learning, game theory principles, and linguistic comprehension allows the discovery of subtle insights through trained simulations.
In summary, trained MARL simulations leverage AI capabilities to uncover insights hidden from limited human analysis. By embracing complexity, strategic reasoning in finance can expand to the next level.
The future of Game Theory with AI
For over 70 years, game theory has provided a useful framework for understanding how strategic decision-makers interact. But applying game theory to real-world investing situations has always been challenging. With so many unknown variables and shifting dynamics, modeling complex market scenarios often exceeds human cognitive capabilities.
New AI techniques are unlocking the potential for sophisticated game theory applications in finance. By combining strategic reasoning, linguistic comprehension, and experiential learning, AI systems can start to account for the intricacies of global markets.
Multi-agent reinforcement learning allows strategic agents to develop and refine techniques through extensive simulation and practice. And large language models like GPT-4 comprehend the nuanced narratives that drive negotiations and relationships between market participants.
Of course, any application of AI requires ethical considerations to avoid issues like bias. But the possibilities are intriguing. Instead of simplifying situations to fit constrained models, AI can embrace the complexity. We can simulate countless permutations of scenarios that likely have never been encountered before.
The result is fast becoming a democratization of game theory, once reserved for the highly educated academics and policy makers. AI unlocks sophisticated strategic analysis for all investors to consider. It provides a more equal footing to understand and respond to real-world market dynamics.
Game theory AI still has much progress to make. But the integration of different methods provides a promising path. We look forward to seeing principled innovations that apply strategic reasoning in finance. And we’re excited by the potential to use AI for good in making markets more efficient and fair. The future is bright for game theory’s next era powered by AI.
To learn more about how AI is transforming finance, connect with the team at Telescope.